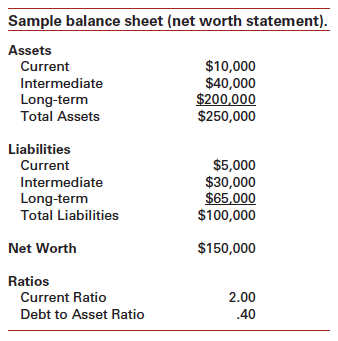
The data are then used in the model as the starting point for a forecast. Commonly, the set of equations used to predict the physics and dynamics of the atmosphere are called primitive equations. These equations are initialized from the analysis data and rates of change are determined.
The rates of change predict the state of the atmosphere a short time into the future. The equations are then applied to this new atmospheric state to find new rates of change, and these new rates of change predict the atmosphere at a yet further time into the future. This time stepping procedure is continually repeated until the solution reaches the desired forecast time. We find this true, for example, in estimating the demand for TV glass by size and customer. In such cases, the best role for statistical methods is providing guides and checks for salespersons’ forecasts.
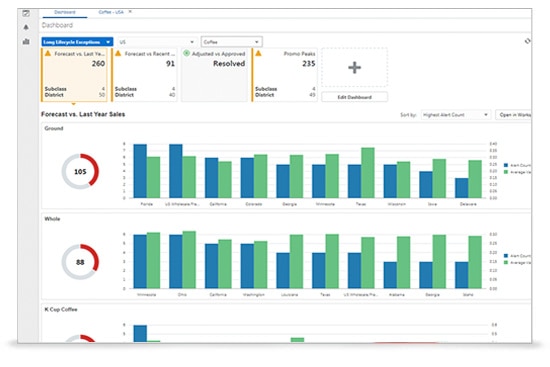
What are the three types of forecasting?
Budgeting quantifies the expectation of revenues that a business wants to achieve for a future period, whereas financial forecasting estimates the number of revenues that will be achieved in a future period.
This graphical representation is what makes time-series data a useful forecasting tool regardless of the time periods involved. Accurate short-term and long-term forecasts are critical to almost every aspect of running a financially successful business. You need accurate forecasts for budgeting, sales revenue estimates, production and inventory, human resources demand planning and purchasing.
The raw output is often modified before being presented as the forecast. This can be in the form of statistical techniques to remove known biases in the model, or of adjustment to take into account consensus among other numerical weather forecasts.
Human input is still required to pick the best possible forecast model to base the forecast upon, which involves pattern recognition skills, teleconnections, knowledge of model performance, and knowledge of model biases. Hence, forecasts become less accurate as the difference between current time and the time for which the forecast is being made (the range of the forecast) increases.
Don’t think it’s about sophisticated financial models or spreadsheets. I was a vice president of a market research firm for several years, doing expensive forecasts, and I saw many times that there’s nothing better than the educated guess of somebody who knows the business well.
Regression Analysis
Examples of qualitative forecasting methods are[citation needed] informed opinion and judgment, the Delphi method, market research, and historical life-cycle analogy. The costs of using these techniques will be reduced significantly; this will enhance their implementation. We expect that computer timesharing companies will offer access, at nominal cost, to input-output data banks, broken down into more business segments than are available today. The continuing declining trend in computer cost per computation, along with computational simplifications, will make techniques such as the Box-Jenkins method economically feasible, even for some inventory-control applications. Computer software packages for the statistical techniques and some general models will also become available at a nominal cost.
An organization uses a variety of forecasting models to assess possible outcomes for the company. The methods used by an individual organization will depend on the data available and the industry in which the organization operates. The primary advantage of forecasting is that it provides the business with valuable information that the business can use to make decisions about the future of the organization.
Qualitative forecasting techniques are generally more subjective than their quantitative counterparts. Qualitative techniques are more useful in the earlier stages of the product life cycle, when less past data exists for use in quantitative methods. Qualitative methods include the Delphi technique, Nominal Group Technique (NGT), sales force opinions, executive opinions, and market research.
In matters of accuracy, the ability to correctly model demand expectations and account both for random variations and unforeseen events, regardless of the time frame or number of different periods, is vitally important. In this direct method of sales forecasting, the marketing manager takes the opinion of the users of a product.
Business Forecasting: Understanding the Basics
For example, GMDH neural network was found to have better forecasting performance than the classical forecasting algorithms such as Single Exponential Smooth, Double Exponential Smooth, ARIMA and back-propagation neural network. In this method, experts in the particular field are requested to give their views on the likely demand for the product in future. Here, a seminar is organized and all the executives of the enterprise participate in this seminar. The opinions of all the executives regarding demand of the product of the enterprise are collected and forecasts are determined on the basis of such opinion. This method is also called the hunch method since the experts give their opinion after weighing pros & cons of all factors affecting the product demand and arrive at an estimate.
Quantitative forecasting models are used to forecast future data as a function of past data. They are appropriate to use when past numerical data is available and when it is reasonable to assume that some of the patterns in the data are expected to continue into the future. These methods are usually applied to short- or intermediate-range decisions. Examples of quantitative forecasting methods are[citation needed] last period demand, simple and weighted N-Period moving averages, simple exponential smoothing, poisson process model based forecasting and multiplicative seasonal indexes. Previous research shows that different methods may lead to different level of forecasting accuracy.
Time series analysis
- Don’t think it’s about sophisticated financial models or spreadsheets.
- I was a vice president of a market research firm for several years, doing expensive forecasts, and I saw many times that there’s nothing better than the educated guess of somebody who knows the business well.
Humans can use knowledge of local effects that may be too small in size to be resolved by the model to add information to the forecast. While increasing accuracy of forecast models implies that humans may no longer be needed in the forecast process at some point in the future, there is currently still a need for human intervention. The forecasting of the weather within the next six hours is often referred to as nowcasting. In this time range it is possible to forecast smaller features such as individual showers and thunderstorms with reasonable accuracy, as well as other features too small to be resolved by a computer model. A human given the latest radar, satellite and observational data will be able to make a better analysis of the small scale features present and so will be able to make a more accurate forecast for the following few hours.
Using the right budgeting and forecasting techniques is essential in creating accurate, reliable forecasts for your business. Decide on your goals, which will lead you to the best forecast tools and techniques to help you start planning and building your strategies to accomplish those goals. There are several business forecasting methods that might work for your business that will help you understand past trends and potential demand. Here are some of the general forecasting models often used by businesses. Essentially, forecasting lets a business look at past trends plus their current position and predict a future.
How to Choose the Right Forecasting Technique
Although the X-11 was not originally developed as a forecasting method, it does establish a base from which good forecasts can be made. One should note, however, that there is some instability in the trend line for the most recent data points, since the X-11, like virtually all statistical techniques, uses some form of moving average. It has therefore proved of value to study the changes in growth pattern as each new growth point is obtained.
You can use business forecast tools to help predict sales, budgets, and more. Having an accurate picture of your business’s potential using data and market trends can help you set and meet objectives. Here are some of the fundamental forecasting tools and techniques to help you plan and strategize effectively. A time series is a useful forecasting method for tracking things such as consumer demand, earnings, profits, shipments, accidents, output and productivity. Time-series forecasting assumes that past behaviors, such as seasonality, trends and cycles, predict future behaviors.
In it, buyers are asked about their future buying intentions of the product, their brand preferences, increase in the prices of products, change in the product’s features, quantities of purchases, etc. Thus, the total sales forecasts of the products are estimated by combining the responses of consumers. Time-series forecasting is a popular quantitative forecasting technique, in which data is gathered over a period of time to identify trends.
Time-series methods are one of the simplest methods to deploy and can be quite accurate, particularly over the short term. Some techniques that fall within this method are simple averaging and exponential smoothing. In the past, the human forecaster was responsible for generating the entire weather forecast based upon available observations. Today, human input is generally confined to choosing a model based on various parameters, such as model biases and performance. Using a consensus of forecast models, as well as ensemble members of the various models, can help reduce forecast error.
In many cases forecasting uses qualitative data that depends on the judgment of experts. While it’s not a crystal ball, forecasting methods can help to assess information for your business’s future.
The use of ensembles and model consensus help narrow the error and pick the most likely outcome. Limitations pose barriers beyond which forecasting methods cannot reliably predict. Events such as the roll of a die or the results of the lottery cannot be forecast because they are random events and there is no significant relationship in the data. Qualitative forecasting techniques are subjective, based on the opinion and judgment of consumers and experts; they are appropriate when past data are not available.
However, there are now expert systems using those data and mesoscale numerical model to make better extrapolation, including evolution of those features in time. Accuweather is known for a Minute-Cast, which is a minute-by-minute precipitation forecast for the next two hours. Once calculated by hand based mainly upon changes in barometric pressure, current weather conditions, and sky condition or cloud cover, weather forecasting now relies on computer-based models that take many atmospheric factors into account.
MOS or model output statistics is a technique used to interpret numerical model output and produce site-specific guidance. This guidance is presented in coded numerical form, and can be obtained for nearly all National Weather Service reporting stations in the United States. As proposed by Edward Lorenz in 1963, long range forecasts, those made at a range of two weeks or more, are impossible to definitively predict the state of the atmosphere, owing to the chaotic nature of the fluid dynamics equations involved. In numerical models, extremely small errors in initial values double roughly every five days for variables such as temperature and wind velocity. The irregularly spaced observations are processed by data assimilation and objective analysis methods, which perform quality control and obtain values at locations usable by the model’s mathematical algorithms (usually an evenly spaced grid).
However, regardless how small the average error becomes with any individual system, large errors within any particular piece of guidance are still possible on any given model run. Humans are required to interpret the model data into weather forecasts that are understandable to the end user.
What is budgeting and forecasting in accounting?
Forecasting in accounting refers to the process of using current and historic cost data to predict future costs. Forecasting is important for planning purposes – it is necessary to estimate and plan for costs that will be incurred prior to actually incurring them.
After collecting time-series data, you display the relationship between time and the variable using a time-series plot. Time is on the horizontal axis and the time-series variable is on the vertical axis.
Thus, on the basis of knowledge and experience of marketing executives, the sales forecasting of the enterprise takes place. The length of the time step chosen within the model is related to the distance between the points on the computational grid, and is chosen to maintain numerical stability. Time steps for global models are on the order of tens of minutes, while time steps for regional models are between one and four minutes. The visual output produced by a model solution is known as a prognostic chart, or prog.