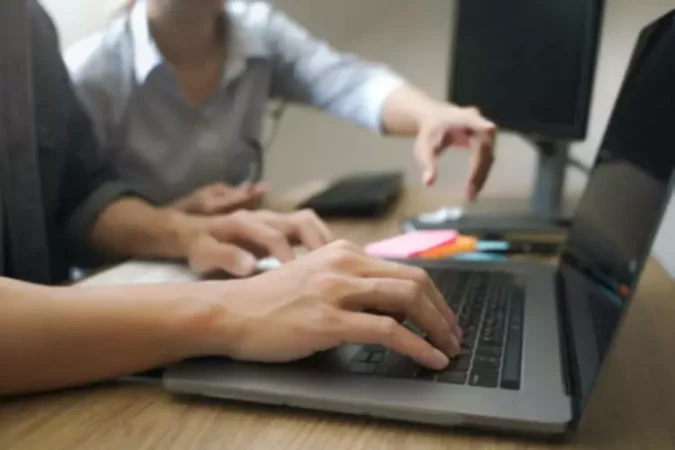
In regression analysis, model building is the process of developing a probabilistic model that best describes the relationship between the dependent and independent variables. The major issues are finding the proper form (linear or curvilinear) of the relationship and selecting which independent variables to include. In building models it is often desirable to use qualitative as well as quantitative variables. The analysis of residuals plays an important role in validating the regression model. If the error term in the regression model satisfies the four assumptions noted earlier, then the model is considered valid.
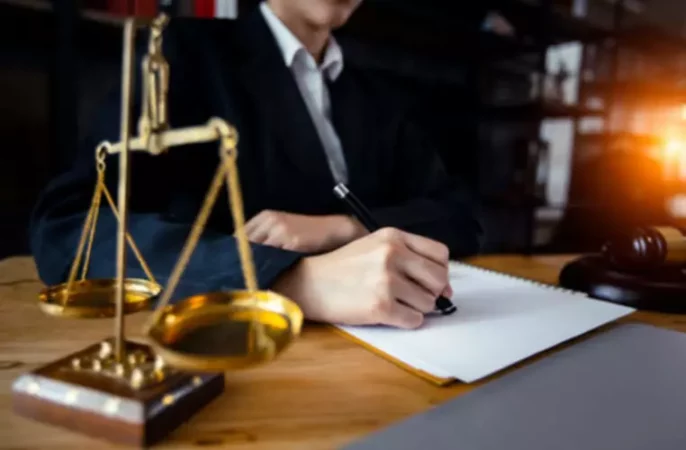
By varying the assumed percentage of defective items in a lot, several different sampling plans can be evaluated and a sampling plan selected such that both the producer’s and consumer’s risks are reasonably low. A primary concern of time series analysis is the development of forecasts for future values of the series. For instance, the federal government develops forecasts of many economic time series such as the gross domestic product, exports, and so on. A time series is a set of data collected at successive points in time or over successive periods of time. A sequence of monthly data on new housing starts and a sequence of weekly data on product sales are examples of time series. Usually the data in a time series are collected at equally spaced periods of time, such as hour, day, week, month, or year.
Nonparametric methods
In these cases, the initial equation is considered as well-posed; and the residual can be considered as a measure of deviation of the approximation from the exact solution. This difference between n and n − 1 degrees of freedom results in Bessel’s correction for the estimation of sample variance of a population with unknown mean and unknown variance. All that we must do is to subtract the predicted value of y from the observed value of y for a particular x.
- A sequence of monthly data on new housing starts and a sequence of weekly data on product sales are examples of time series.
- These residuals, computed from the available data, are treated as estimates of the model error, ε.
- The most common residual plot shows ŷ on the horizontal axis and the residuals on the vertical axis.
- Correct decisions correspond to accepting a good-quality lot and rejecting a poor-quality lot.
- The straight line that best fits that data is called the least squares regression line.
The Wilcoxon signed-rank test can be used to test hypotheses about two populations. In collecting data for this test, each element or experimental unit in the sample must generate two paired or matched data values, one from population 1 and one from population 2. Differences between the paired or matched data values are used to test for a difference between the two populations. The Wilcoxon signed-rank test is applicable when no assumption can be made about the form of the probability distributions for the populations.
Score for RESIDUAL
In regression analysis, the distinction between errors and residuals is subtle and important, and leads to the concept of studentized residuals. Given an unobservable function that relates the independent variable to the dependent variable – say, a line – the deviations of the dependent variable observations from this function are the unobservable errors. If one runs a regression on some data, then the deviations of the dependent variable observations from the fitted function are the residuals. If they are random, or have no trend, but “fan out” – they exhibit a phenomenon called heteroscedasticity. If all of the residuals are equal, or do not fan out, they exhibit homoscedasticity.
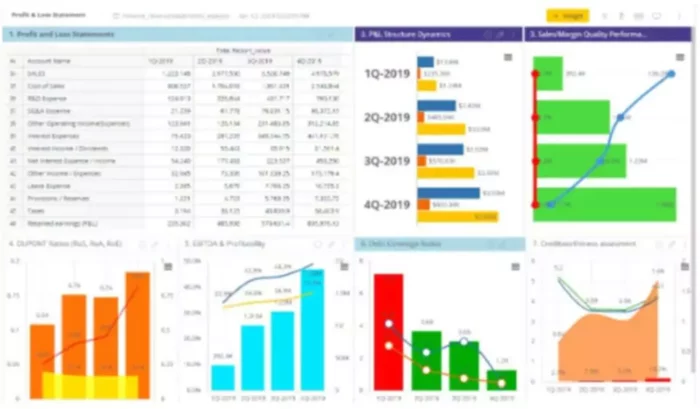
The ith residual is the difference between the observed value of the dependent variable, yi, and the value predicted by the estimated regression equation, ŷi. These residuals, computed from the available data, are treated as estimates of the model error, ε. As such, they are used by statisticians to validate the assumptions concerning ε. Statistical quality control refers to the use of statistical methods in the monitoring and maintaining of the quality of products and services. One method, referred to as acceptance sampling, can be used when a decision must be made to accept or reject a group of parts or items based on the quality found in a sample. A second method, referred to as statistical process control, uses graphical displays known as control charts to determine whether a process should be continued or should be adjusted to achieve the desired quality.
Words for Lesser-Known Games and Sports
A Spearman rank correlation coefficient of 1 would indicate complete agreement, a coefficient of −1 would indicate complete disagreement, and a coefficient of 0 would indicate that the rankings were unrelated. Statistical process control uses sampling and statistical methods to monitor the quality of an ongoing process such as a production operation. Whenever assignable causes are identified, a decision can be made to adjust the process in order to bring the output back to acceptable quality levels.
residual 11 letter words
Because sampling is being used, the probabilities of erroneous decisions need to be considered. The error of rejecting a good-quality lot creates a problem for the producer; the probability of this error is called the producer’s risk. On the other hand, the error of accepting a poor-quality lot creates a problem for the purchaser or consumer; the probability of this error is called the consumer’s risk. Linear regression is a statistical tool that determines how well a straight line fits a set of paired data. The straight line that best fits that data is called the least squares regression line.
Likewise, the sum of absolute errors (SAE) is the sum of the absolute values of the residuals, which is minimized in the least absolute deviations approach to regression. Thus to compare residuals at different inputs, one needs to adjust the residuals by the expected variability of residuals, which is called studentizing. This is particularly important in the case of detecting outliers, where the case in question is somehow different from the others in a dataset. For example, a large residual may be expected in the middle of the domain, but considered an outlier at the end of the domain. For instance, an x̄-chart is employed in situations where a sample mean is used to measure the quality of the output.
A statistical error (or disturbance) is the amount by which an observation differs from its expected value, the latter being based on the whole population from which the statistical unit was chosen randomly. The expected value, being the mean of the entire population, is typically unobservable, and hence the statistical error cannot be observed either. The design of an acceptance sampling plan consists of determining a sample size n and an acceptance criterion c, where c is the maximum number of defective items that can be found in the sample and the lot still be accepted. The key to understanding both the producer’s risk and the consumer’s risk is to assume that a lot has some known percentage of defective items and compute the probability of accepting the lot for a given sampling plan.