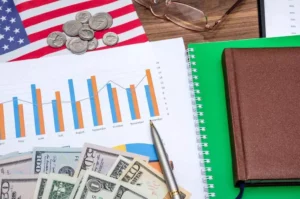
Variance is essentially the degree of spread in a data set about the mean value of that data. It shows the amount of variation that exists among the data points. Visually, the larger the variance, the “fatter” a probability distribution will be. In finance, if something like an investment has a greater variance, it may be interpreted as more risky or volatile. Yes, ANOVA tests assume that the data is normally distributed and that the levels of variance in each group is roughly equal.
Adding the two variables together, we get an overall variance of $4,800 (Unfavorable). Management should address why the actual labor price is a dollar higher than the standard and why 1,000 more hours are required for production. The same column method can also be applied to variable overhead costs. It is similar to the labor format because the variable overhead is applied based on labor hours in this example.
Fixed Overhead Variance
The analyst utilizes the ANOVA test results in an f-test to generate additional data that aligns with the proposed regression models. Follow-up tests to identify which specific groups, variables, or factors have statistically different means include the Tukey’s range test, and Duncan’s new multiple range test. In turn, these tests are often followed with a Compact Letter Display (CLD) methodology in order to render the output of the mentioned tests more transparent to a non-statistician audience.
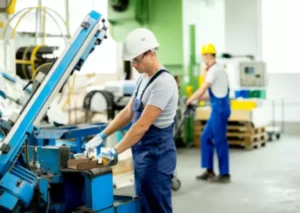
Adding these two variables together, we get an overall variance of $3,000 (unfavorable). It is a variance that management should look at and seek to improve. Although price variance is favorable, management may want to consider why the company needs more materials than the standard of 18,000 pieces.
How to Calculate Variance Calculator, Analysis & Examples
However, the significant overlap of distributions, for example, means that we cannot distinguish X1 and X2 reliably. Grouping dogs according to a coin flip might produce distributions that look similar. Uneven variances between samples result in biased and skewed test results. If you have uneven variances across samples, non-parametric tests are more appropriate. When you collect data from a sample, the sample variance is used to make estimates or inferences about the population variance.
- The main idea behind an ANOVA is to compare the variances between groups and variances within groups to see whether the results are best explained by the group differences or by individual differences.
- Often, by analyzing these variances, companies are able to use the information to identify a problem so that it can be fixed or simply to improve overall company performance.
- In statistics, variance measures variability from the average or mean.
- However, the variance is more informative about variability than the standard deviation, and it’s used in making statistical inferences.
- Different formulas are used for calculating variance depending on whether you have data from a whole population or a sample.
This is actually a group of distribution functions, with two characteristic numbers, called the numerator degrees of freedom and the denominator degrees of freedom. A statistically significant effect in ANOVA is often followed by additional tests. This can be done in order to assess which groups are different from which other groups or to test various other focused hypotheses. There are three classes of models used in the analysis of variance, and these are outlined here.
Population vs. sample variance
Over 1.8 million professionals use CFI to learn accounting, financial analysis, modeling and more. Start with a free account to explore 20+ always-free courses and hundreds of finance templates and cheat sheets. Divide the sum of the squares by n – 1 (for a sample) or N (for a population). ANOVA is a good way to compare more than two groups to identify relationships between them. The technique can be used in scholarly settings to analyze research or in the world of finance to try to predict future movements in stock prices. Understanding how ANOVA works and when it may be a useful tool can be helpful for advanced investors.
It is utilized to observe the interaction between the two factors and tests the effect of two factors at the same time. The t- and z-test methods developed in the 20th century were used for statistical analysis until 1918, when Ronald Fisher created the analysis of variance method. ANOVA is also called the Fisher analysis of variance, and it is the extension of the t- and z-tests. The term became well-known in 1925, after appearing in Fisher’s book, “Statistical Methods for Research Workers.” It was employed in experimental psychology and later expanded to subjects that were more complex.
- We can consider the 2-way interaction example where we assume that the first factor has 2 levels and the second factor has 3 levels.
- It is utilized to observe the interaction between the two factors and tests the effect of two factors at the same time.
- If you have uneven variances across samples, non-parametric tests are more appropriate.
- The square root of the variance is the standard deviation (SD or σ), which helps determine the consistency of an investment’s returns over a period of time.
- Users often employ it primarily to take the square root of its value, which indicates the standard deviation of the data.
- Access and download collection of free Templates to help power your productivity and performance.
The main idea behind an ANOVA is to compare the variances between groups and variances within groups to see whether the results are best explained by the group differences or by individual differences. The variance is usually calculated automatically by whichever software you use for your statistical analysis. But you can also calculate it by hand to better understand how the formula works. When you have collected data from every member of the population that you’re interested in, you can get an exact value for population variance. The standard deviation is derived from variance and tells you, on average, how far each value lies from the mean.
Step 4: Find the sum of squares
Since the randomization-based analysis is complicated and is closely approximated by the approach using a normal linear model, most teachers emphasize the normal linear model approach. Few statisticians object to model-based analysis of balanced randomized experiments. The analysis of variance has been studied from several approaches, the most common of which uses a linear model that relates the response to the treatments and blocks.
Homogeneity of variance in statistical tests
If these assumptions are not accurate, ANOVA may not be useful for comparing groups. Reporting sample size analysis is generally required in psychology. In the design of an experiment, the number of experimental units is planned to satisfy the goals of the experiment. Variance is important to consider before performing parametric tests.
Summary of assumptions
The assumption of unit treatment additivity usually cannot be directly falsified, according to Cox and Kempthorne. However, many consequences of treatment-unit additivity can be falsified. For a randomized experiment, the assumption of unit-treatment additivity implies that the variance is constant for all treatments. Therefore, by contraposition, a necessary condition for unit-treatment additivity is that the variance is constant.