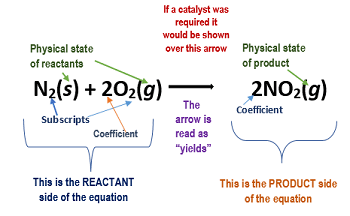
Negative life events and depression were found to be the strongest predictors of youth aggression. Another assumption of multiple regression is that the X variables are not multicollinear.
In other terms, MLR examines how multiple independent variables are related to one dependent variable. Once each of the independent factors has been determined to predict the dependent variable, the information on the multiple variables can be used to create an accurate prediction on the level of effect they have on the outcome variable.
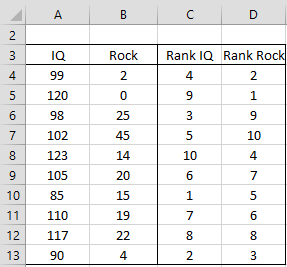
It also assumes that each independent variable would be linearly related to the dependent variable, if all the other independent variables were held constant. This is a difficult assumption to test, and is one of the many reasons you should be cautious when doing a multiple regression (and should do a lot more reading about it, beyond what is on this page). It is easy to throw a big data set at a multiple regression and get an impressive-looking output. However, many people are skeptical of the usefulness of multiple regression, especially for variable selection.
Third, multiple linear regression assumes that there is no multicollinearity in the data. No Multicollinearity—Multiple regression assumes that the independent variables are not highly correlated with each other. This assumption is tested using Variance Inflation Factor (VIF) values. The second table contains the coefficients, their standard errors, test statistic (t), p-values, and 95% confidence interval, for each predictor variable in the model, grouped by outcome.
Setup in SPSS Statistics
This means that different researchers, using the same data, could come up with different results based on their biases, preconceived notions, and guesses; many people would be upset by this subjectivity. Whether you use an objective approach like stepwise multiple regression, or a subjective model-building approach, you should treat multiple regression as a way of suggesting patterns in your data, rather than rigorous hypothesis testing. Atlantic beach tiger beetle, Cicindela dorsalis dorsalis.One use of multiple regression is prediction or estimation of an unknown Y value corresponding to a set of X values. For example, let’s say you’re interested in finding suitable habitat to reintroduce the rare beach tiger beetle, Cicindela dorsalis dorsalis, which lives on sandy beaches on the Atlantic coast of North America. Multiple regression would give you an equation that would relate the tiger beetle density to a function of all the other variables.
Test Procedure in SPSS Statistics
However, many people just call them the independent and dependent variables. More advanced regression techniques (like multiple regression) use multiple independent variables. A simple linear regression is a function that allows an analyst or statistician to make predictions about one variable based on the information that is known about another variable. Linear regression can only be used when one has two continuous variables—an independent variable and a dependent variable. The independent variable is the parameter that is used to calculate the dependent variable or outcome.
Correlation and Regression are the two analysis based on multivariate distribution. A multivariate distribution is described as a distribution of multiple variables. Correlation is described as the analysis which lets us know the association or the absence of the relationship between two variables ‘x’ and ‘y’. On the other end, Regression analysis, predicts the value of the dependent variable based on the known value of the independent variable, assuming that average mathematical relationship between two or more variables. Multiple linear regression (MLR), also known simply as multiple regression, is a statistical technique that uses several explanatory variables to predict the outcome of a response variable.
The goal of multiple linear regression (MLR) is to model the linear relationship between the explanatory (independent) variables and response (dependent) variable. Multiple regression is used to examine the relationship between several independent variables and a dependent variable.
Multivariate analysis examines several variables to see if one or more of them are predictive of a certain outcome. The predictive variables are independent variables and the outcome is the dependent variable. The variables can be continuous, meaning they can have a range of values, or they can be dichotomous, meaning they represent the answer to a yes or no question.
What is Multiple Regression Analysis?
A multiple regression model extends to several explanatory variables. Multivariate analysis was used in by researchers in a 2009 Journal of Pediatrics study to investigate whether negative life events, family environment, family violence, media violence and depression are predictors of youth aggression and bullying.
- Multivariate analysis examines several variables to see if one or more of them are predictive of a certain outcome.
This could help you guide your conservation efforts, so you don’t waste resources introducing tiger beetles to beaches that won’t support very many of them. Multiple regression analysis can be performed using Microsoft Excel and IBM’s SPSS. Other statistical tools can equally be used to easily predict the outcome of a dependent variable from the behavior of two or more independent variables. In business, sales managers use multiple regression analysis to analyze the impact of some promotional activities on sales.
The model creates a relationship in the form of a straight line (linear) that best approximates all the individual data points. Multiple Regressions are a method to predict the dependent variable with the help of two or more independent variables. While running a this analysis, the main purpose of the researcher is to find out the relationship between the dependent variable and the independent variables. In order to predict the dependent variable, multiple independent variables are chosen which can help in predicting the dependent variable.
Multicollinearity occurs when two independent variables are highly correlated with each other. For example, let’s say you included both height and arm length as independent variables in a multiple regression with vertical leap as the dependent variable. Because height and arm length are highly correlated with each other, having both height and arm length in your multiple regression equation may only slightly improve the R2 over an equation with just height. So you might conclude that height is highly influential on vertical leap, while arm length is unimportant. However, this result would be very unstable; adding just one more observation could tip the balance, so that now the best equation had arm length but not height, and you could conclude that height has little effect on vertical leap.
It is used when linear regression is not able to do serve the purpose. Regression analysis helps in the process of validating whether the predictor variables are good enough to help in predicting the dependent variable. You’re probably familiar with plotting line graphs with one X axis and one Y axis. The X variable is sometimes called the independent variable and the Y variable is called the dependent variable.
Simple linear regression plots one independent variable X against one dependent variable Y. Technically, in regression analysis, the independent variable is usually called the predictor variable and the dependent variable is called the criterion variable.
Multicollinearity occurs when the independent variables are too highly correlated with each other. Multiple regressions are based on the assumption that there is a linear relationship between both the dependent and independent variables. It also assumes no major correlation between the independent variables.
What is a multiple regression analysis used for?
Multiple regression is an extension of simple linear regression. It is used when we want to predict the value of a variable based on the value of two or more other variables. The variable we want to predict is called the dependent variable (or sometimes, the outcome, target or criterion variable).
Multiple regression analysis is the most common method used in multivariate analysis to find correlations between data sets. Others include logistic regression and multivariate analysis of variance. Multiple linear regression (MLR) is used to determine a mathematical relationship among a number of random variables.
Multiple linear regression requires at least two independent variables, which can be nominal, ordinal, or interval/ratio level variables. A rule of thumb for the sample size is that regression analysis requires at least 20 cases per independent variable in the analysis. If your goal is prediction, multicollinearity isn’t that important; you’d get just about the same predicted Y values, whether you used height or arm length in your equation. However, if your goal is understanding causes, multicollinearity can confuse you. Before doing multiple regression, you should check the correlation between each pair of independent variables, and if two are highly correlated, you may want to pick just one.
What do you mean by multiple regression analysis?
Definition: Multiple regression analysis is a statistical method used to predict the value a dependent variable based on the values of two or more independent variables.
It is used when we want to predict the value of a variable based on the value of two or more other variables. The variable we want to predict is called the dependent variable (or sometimes, the outcome, target or criterion variable). The variables we are using to predict the value of the dependent variable are called the independent variables (or sometimes, the predictor, explanatory or regressor variables). The first step in finding a linear regression equation is to determine if there is a relationship between the two variables. You’ll also need a list of your data in x-y format (i.e. two columns of data—independent and dependent variables).
A real estate agent could use multiple regression to analyze the value of houses. For example, she could use as independent variables the size of the houses, their ages, the number of bedrooms, the average home price in the neighborhood and the proximity to schools. Plotting these in a multiple regression model, she could then use these factors to see their relationship to the prices of the homes as the criterion variable.
In this case, negative life events, family environment, family violence, media violence and depression were the independent predictor variables, and aggression and bullying were the dependent outcome variables. Over 600 subjects, with an average age of 12 years old, were given questionnaires to determine the predictor variables for each child. Multiple regression equations and structural equation modeling was used to study the data set.
Multiple regression analysis can be used to also unearth the impact of salary increment and increments in other employee benefits on employee output. The analysis is useful when you want to predict the impact of individual independent variables on the desired outcome. The value being predicted is termed dependent variable because its outcome or value depends on the behavior of other variables. The independent variables’ value is usually ascertained from the population or sample. Thirdly, linear regression assumes that there is little or no multicollinearity in the data.
The coefficient of determination (R-squared) is a statistical metric that is used to measure how much of the variation in outcome can be explained by the variation in the independent variables. R2 always increases as more predictors are added to the MLR model even though the predictors may not be related to the outcome variable.